Photo by NASA on Unsplash
Sponsored content from Software AG

As the value of analytics and artificial intelligence (AI) grows, organizations need ways to get a complete view of data across all their applications and databases. This is more challenging than it may first seem because, for most organizations, the future involves a hybrid of on-premises mainframes and the cloud. IBM Z® mainframes deliver reliability, security, and performance for business continuity. New cloud services offer a compelling proposition for innovative applications and on-demand elastic infrastructure.
For these two very different worlds to work together, you need a data integration solution that keeps your cloud data in sync with your legacy systems of record, using minimal resources. The best approach enables you to easily move, sync, and replicate data between on-premises infrastructure and the cloud, so you can make the most of your data wherever it resides, no matter which application needs it.
Emerging analytics capabilities are becoming cloud-first and cloud-only. Gartner projects that by 2022, public cloud services will be essential for 90% of data and analytics innovation (a topic to be covered during Gartner’s upcoming Data & Analytics Summit). This means it is critical to connect your mainframe to the cloud — and cloud to mainframe — so all your data can inform the analytics, BI, machine learning, and AI tools that will power future success.
Cloud and mainframe, working together
While cloud applications and databases grow by leaps and bounds, organizations find compelling reasons to keep their on-premises systems. Between 40–80% of enterprise data either resides or originates on the mainframe, and some 70% of Fortune 500 businesses use mainframes for core operations because they offer fast, reliable performance, with decades of investment in unique business logic. It’s hard to overstate the value of business continuity and trusted security.
And then there is the cloud: elastic, scalable, and continuously improving. Cloud application platforms are increasingly attractive, and important: Bain & Company has found that CIOs already consider their cloud service providers to be critical, strategic IT partners. The average company uses 53 cloud platform services, beyond basic computing and storage.
Cloud is also rising to the challenge of the data explosion. IDC forecasts that more data will be created over the next three years than was created over the past three decades — much of it will reside in elastic data warehouses and cloud data lakes that open new capabilities for business intelligence, analytics, and artificial intelligence.
Integration — the ability to move, sync, and replicate data across a range of systems — is the secret to a seamless connection across mainframe, big data, and the cloud. It is how you can gain the analytics benefits of moving your data warehouse to the cloud while keeping your diverse data sources in sync.
A closer look at hybrid data movement
The right integration strategy is a critical component of an AI-ready, data-centric organization. In a seamless hybrid world, you can push data from on-premises operations up to the cloud to generate insights that lead to better customer services and greater innovation. And, you can pull data from applications in the cloud to update your on-premises systems of record.
Consider an increasingly common scenario: copying data from the mainframe to a cloud data warehouse or data lake. Data lakes, such as Snowflake, are valuable for efficient consolidation, access, and reporting, as well as the cloud’s ever-growing BI and analytics capabilities. You need to ensure your data in the cloud is reliable and up-to-date without compromising the on-premises source systems’ uptime and performance. This means replicating your enterprise data to the cloud, ideally with the option to capture only changed data so you’re not using service unit resources to update static information.
The other direction is propagating data from cloud to the mainframe. When your organization adopts a new cloud application, you need to forward the data to the mainframe database, your system of record. Or, in the case of mainframe modernization, if you replace one module of a mainframe application with a new cloud application, the mainframe will need to receive data from the cloud.
For this hybrid model to work smoothly, you need an integration solution that helps you overcome four common challenges:
- Connectivity: a method that connects to all your data sources on mainframe, midrange, desktop, and cloud platforms
- Latency: a method to bring together a world of varied data structures on a data warehouse or data lake fast enough to enable real-time dashboarding, analytics, and streaming analytics
- Transformation: a method to transform data in flight beyond simple extract, transform, load (ETL) processes, both to change metadata and to support compatibility between cloud-based data warehouses, diverse data formats and non-relational data sources
- Simplicity: a single, reliable, cost-effective solution to connect data across cloud and on-premises environments, that is easy to implement and update
Organizations looking for such integration encounter service providers with a range of experience and capabilities. Broadly speaking, there is a divide between pure-play data integration and platform vendors, the latter offering a range of capabilities that often include data quality, data governance, and data cataloguing. From our long experience in the market, I can say with confidence that pure-play integration vendors often out-compete platforms by offering greater value, greater flexibility and superior performance for the capabilities and use cases that matter most to you. Indeed, Bloor Research International recently took a fresh look at the trends around data integration, and found that pure-play vendors can typically claim “significant total cost of ownership benefits” when compared to broader platform vendors.
It may sound obvious, but the best integration system is one that meets your needs: complete and right-sized.
Complete means out-of-the box connectivity to all your data sources and platforms, including mainframe and any other legacy data sources you still rely on. It should have full extract, transform, load (ETL) and extract, load, transform (ELT) features to transform data in flight, with comprehensive ways to map tables and fields. For integration between mainframe and cloud, your solution should have features to incrementally update only those records that have changed, reducing bandwidth and workload on your on-premises platforms. And complete means features for data access, data virtualization, and data movement, so you don’t need to add overhead and complexity by piecing together multiple technologies.
Right-sized means you pay for the capabilities you need, and not a bundle of unnecessary features.
With the power of efficient data movement, real-time data replication and near real-time synchronization, your enterprise and cloud systems can work together seamlessly. To show what this looks like in action, Software AG has shared a webinar with live demos of hybrid data movement between the mainframe and the cloud. By combining the strengths of your mainframe and the innovation on the cloud, you can build a hybrid future that works.
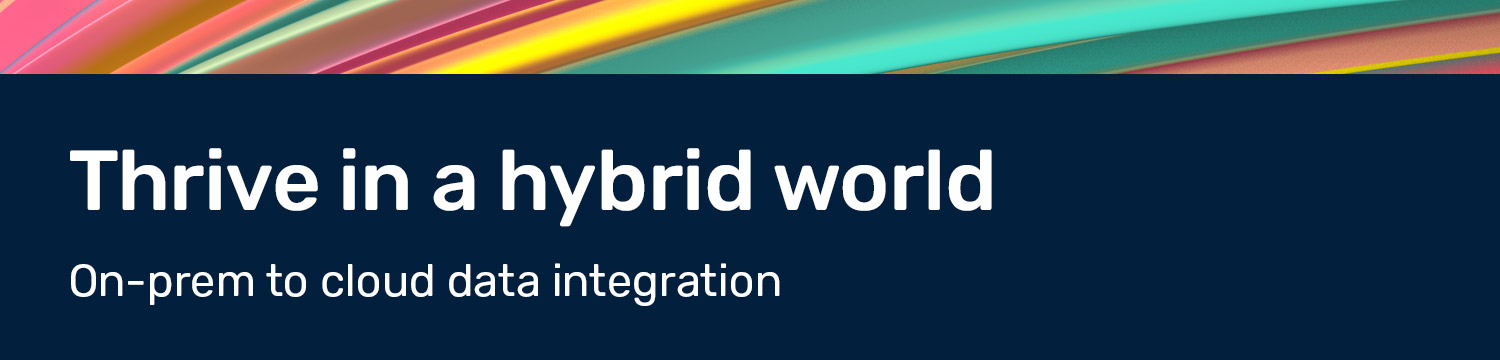
Harpal Gill, vice president of CONNX at Software AG, is responsible for product strategy, sales and worldwide alliances for the company’s data integration solution CONNX. CONNX provides a full-featured suite of data access, virtualization and data movement tools that provide secure, real-time access to more than 150+ data sources — regardless of location. Harpal has a long history of developing strategic partnerships with established and new companies to secure the broadest reach of data source integrations with CONNX. With more than 25 years of experience in business intelligence, data access and visualization, ETL and SaaS domains, Harpal understands the value of data and what it can do for your business.